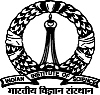 |
Modelling and Visualization of Urban Trajectory in 4 cities of India
T.V. Ramachandra1, Bharath H Aithal2, Vinay S3, Joshi N V4, Uttam Kumar5, Venugopal Rao K6
|
 |
1Energy & Wetlands Research Group, Centre for Ecological Sciences,
Indian Institute of Science, Bangalore. cestvr@ces.iisc.ernet.in
2 Research Associate at Indian Institute of Science (IISc). bharath@ces.iisc.ernet.in 3research scholar at Energy and Wetlands Research Group (EWRG), Centre for Ecological Sciences (CES), Indian Institute of Science (IISc). vinay@ces.iisc.ernet.in 4Faculty at Centre for Ecological Sciences (CES), Indian Institute of Science (IISc), Bangalore 5currently Post-doctoral fellow at NASA AMES Research Centre, USA 6Group Head, Urban Studies & Geoinformatics, National Remote Sensing Centre (NRSC), Indian
Data and Methods
Three phase process involved in simulating the landscape transitions are depicted in Figure 2, which include i) Data Acquisition and Feature Extraction, ii) Land use analysis, iii) Land scape modeling and visualization.
Data acquisition involves acquiring Optical (Landsat series from USGS, http://www.usgs.gov and IRS series, http://www.nrsc.gov.in) and Radar (SRTM DEM from USGS) satellite data, the Survey of India (http://survayofindia.gov.in) Topographical maps (1:50000 and 1:250000 scale), Field data using pre calibrated GPS, City maps, Cities Comprehensive development plans (CDP), and other supporting secondary data such as online spatial portals - Google Earth (http://earth.google.com), Bhuvan (http://bhuvan.nrsc.gov.in) and ancillary databases. Optical satellite data is subjected to radiometric enhancements and geometric corrections with help of secondary data, Field data and Topographic maps. Whereas the radar data is used to extract maps such as DEM, Slope and Drainage networks which play a crucial role in modeling. City administrative boundary is extracted using Topographic maps and supporting datasets. Based on the city boundary, 10 km buffer zone (circular) is delineated. Factors supporting the growth namely road network, bus stops, railway stations, educational institutes, industries and other important socio economic places, using Google earth. Constraints for growth are derived from the CDP maps which include Protected regions (Reserve forests, valley zones, parks…. Etc.) and defense establishments. Both factors and constraints for development for the study sites are presented in the annexures (annexure 1-4).
Land use analysis was carried out using the supervised classifier - Gaussian Maximum Likelihood Classifier (GMLC). The process involved, i) Preparation of False Colour Composites to locate heterogeneous patches, ii) Collection of training data sets (based on heterogeneous patches in FCC) from field using GPS supplemented by Google Earth and Bhuvan virtual spatial databases, covering at least 15% of the study area, iii) GMLC was used to for classification, where in 60% of the training data are used for land use analysis and 40% of the training data are used for accuracy assessment. The land use data of various time frames were used to understand future landscapes based on the current dynamics.
Landscape prediction model was built by integrating various concepts namely Markov chains, Fuzzy logic, Analytical hierarchical process, Multi criteria evaluation and Cellular automata. Markov chains were used to understand the transition of various landscapes between two time periods with respect to which future land use transition areas and probabilities are calculated. Effect up to which factor can contribute to dynamics was determined and normalized between 0 to 255 using the fuzzy approach considering sigmoidal increase or decrease function with respect to land use category. Analytical hierarchical process with the help of expert’s opinion was used to understand the importance of one factor over the other and to derive weightage of each factor that contributes to the dynamics. Calibrations of weights were based on the consistency ratio. Model with lower consistency ratio i.e., less than 0.1 was considered to be consistent and used for further processes. Site suitability maps were developed using multi criteria evaluation techniques based on weighted linear combination of weights for each of the factors derived from AHP process and constraints derived as Boolean (0 – no alterations, 1- can alter). Site suitability maps along with the Markov transition areas are used in combination to simulate the land use using Cellular automata. Cellular automata consider neighboring pixels and transition areas to predict/simulate land use of the next time period. The simulated output along with the land use for the simulated year is compared and validated. Based on the accuracy of the model, the model is subjected to calibration and further Predictions area carried out up to the year 2030 with suitable time steps.
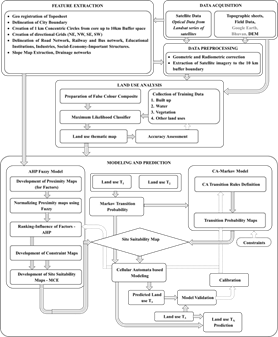
Fig. 2. Method involved
Citation:Ramachandra T. V., Bharath H. Aithal, Vinay S,, Uttam Kumar, Venugopal Rao K and Joshi N V, 2016. Modelling and Visualization of Urban Trajectory in 4 cities of India, 32nd Annual in-house Symposium on Space science and Technology, ISRO-IISc-STC, 7-8 January 2016
|